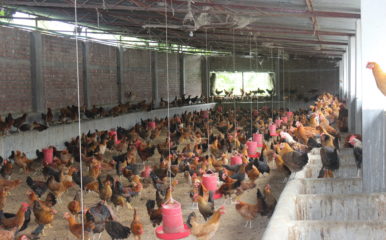
Poultry, pathogens and place – why we need to make predictions
Published on 02/10/2024
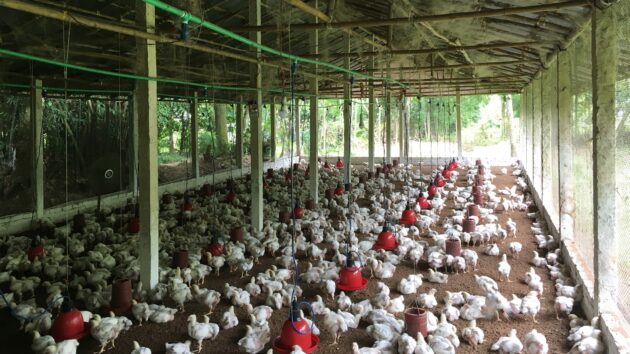
In an ideal world, detailed data on livestock farms, including poultry, farms, would be comfortably sitting in some database that is easily accessible by researchers. Such data is critical to assess the environmental impact of meat production and the risk of diseases spreading through livestock populations.
In practice, however, an accurate census of farm locations is typically unavailable in many countries.
Collecting such information requires time and resources. These costs are simply too high for most low- and middle-income countries (LMICs) such as Bangladesh, India and Vietnam where we have been working.
Another practical challenge with LMICs is that their poultry sectors are rapidly evolving under our eyes: any accurate farm census snapshot would become outdated in a matter of years. Farm distributions represent not just a difficult target, but also a fast-moving one.
In any case, and in all countries including high-income ones, privacy concerns limit access to data.
However, mathematical modelling can come to the rescue!
Statistical models
Challenges in data collection prompted researchers in the One Health Poultry Hub to seek alternative routes to characterise distributions of poultry farms – that is, where farms are located and how far away from each other. One solution we devised involves the use of statistical models to predict farm locations.
The result is a collection of geo-referenced points (the farms) that replaces the real but unobservable farm distribution. This novel Farm Distribution Model (FDM), developed by our team led by Marie-Cécile Dupas, falls in this category and it promises to overcome the limitations of existing models.
Indeed, the FDM is able to capture some of the finer spatial details of real farm distributions such as clustering. Spatial clustering (in short) is the tendency of farms to lump in space.
In addition, the FDM can also predict farm sizes via a dedicated statistical routine.
Epidemic spread
We used the synthetic farm distributions generated by the FDM as an input into an epidemic simulator. The latter enables generating outbreaks of a hypothetical pathogen ripping through a population of farms whose locations are specified by the user. The likelihood of spread between an infected and a susceptible farm depends on their mutual distance. In this manuscript we considered the emergence of a rapidly spreading, avian flu-like virus.
The same computer experiment was repeated using actual farm distributions available for our research sites in Gujarat in India, Bangladesh and Vietnam, and the results were compared with simulations using FDM surrogate (i.e., computer-generated) distributions.
We found that in each site the surrogate distributions yielded good estimates of epidemic risk (defined as the systemic vulnerability of farms to large outbreaks). Interestingly, purely random spatial distributions of farms severely underestimated epidemic risk, further underscoring the importance of accounting for spatial clustering.
Policy implications
The FDM model enables an accurate mapping of poultry farms. These maps are key to better understanding the risk of future epidemics originating on farms. It can also be pivotal for understanding the environmental impact of farms in specific areas.
The algorithm for the FCD is open-source and the team is currently working on ways in which it can be made accessible to policymakers. They will then be able to use it to consider varying scenarios to inform public health responses. The framework will be particularly useful for LMICs where data is scarce and hard to come by and where the rapid intensification process is transforming the landscape of poultry production.